Imagine a future where you have an artificial intelligence (AI) assistant that can help you with all your daily tasks. Whether that might be in the form of responding to people that you don’t like or generating original content (such as art, movies, and video games) personalized for you. You wake up on a rainy day and your AI has a brand-new personalized movie made just for you. All you want to do is just relax, so you turn on auto-respond mode, which knows your vernacular and will respond to any notifications you get while watching your movie.
While this future is not all here yet, we are getting close. Within the last decade, a concept called reinforcement learning is becoming more important to the advancement of AI. In reinforcement learning the AI uses itself to train a model to do something by trial and error, without the need of a human to iteratively make it better [1]. The process works in such mysterious ways that researchers found that it is basically impossible to reverse engineer it to find out how the model reached a certain output. Scientists discovered that using reinforcement learning this way has made a huge improvement to how AI models predict data [1].Reinforcement learning has enabled the creation of some very interesting generative AI models, which can generate content for you. The most common generative model that you have probably heard of is the Deepfake model, where a person’s face can be mapped to another person. However, this was just the beginning for generative models. Nowadays, the generated content can include images, human speech, and even stories [2]–[7]. These models have provided some of the most interesting advancements in the field of artificial intelligence to date, which is why they might be the key to creating a general-purpose AI that can handle many different tasks. This is trying to raise the question; if AI can do all this right now, how long until it can do almost everything a human can?
Invention of Generative Models
The invention of generative models in reinforcement learning is one of the most important breakthroughs in the field of AI to date. In a paper authored by Ian Goodfellow in 2014, Goodfellow describes a new framework for generative models [8]. One of the key factors implemented by Goodfellow to achieve these models was the use of loss functions, specifically the Mini-Max algorithm, used in two-player turn-based games such as Chess and Tic-Tac-Toe when you want to play against the computer [9]. Goodfellow states that in the future a lot of the improvements to generative models will come from new and better loss functions, which is already happening [10]–[12]. This was a huge advancement in AI and has enabled the creation of many different generative models based on Goodfellows work. The true power is just starting to be uncovered, there really is no limit to the various applications and fields these models could be applied to [12].
Image/Art Generation
Art generation is the first step towards our AI driven future. Currently, this is one of the most notable features of generative models. The creativity in art is something that AI should be naturally opposed to, following logic and data, but in recent years this idea has been proven wrong [3]. If we want AI to be able to create a multitude of things it is going to need to understand creativity. As of now, these models are capable of producing high quality images with a lot of variance and uniqueness in each image [10]. A company called OpenAI have been the pioneers in generated art and are already on their second version of their model. Their open-source approach to AI to made it easy for the general public to see what is going on behind the scenes. OpenAI’s model allows users to add descriptive keywords, and anything that could describe an image really.
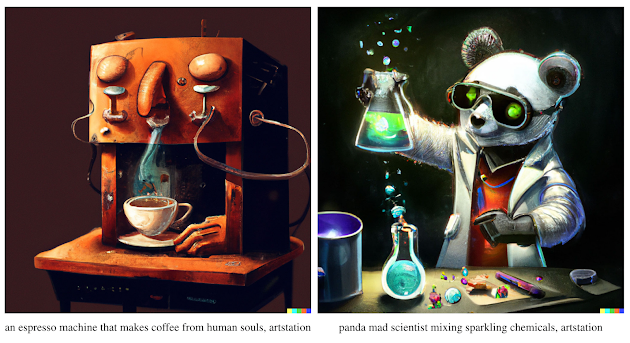
OpenAI’s model seems to understand creativity well, even though it is just a machine trained version of it. In order to test this, researchers conducted experiments to see if people could tell the difference between real art and generated art and found that they couldn’t do it [3]. The generated art was too like human art for regular people to be able to tell the difference. In contrast, the art world believes that these images have “no soul”, “no emotion”, and their perceived creativity is just trained creativity stolen from real artists, because these models are trained on countless human art data. The scientific field also seems to think AI still doesn’t understand what makes “good” art, which will be crucial in the future to generate more kinds of content [3]. So it seems like our AI generated movie is still some ways off [10]. Image generation will play a very important role in the creation of general-AI, as these models will be how the AI sees the world. OpenAI’s art model is truly revolutionary, it shows how far image generation has come in such little time, which makes you wonder if a general-purpose AI may not be as far off as previously thought.
Human-like Speech Generation
OpenAI also has the most mind-blowing applications with human-like speech and text. They have created a general-purpose text-in, text-out AI model [4]. It’s their third version of this model, which brought huge improvements to previous versions, and it has shown no signs of improvement stagnation. This isn’t just an advanced spell check; it can understand concepts and situations and respond accordingly. It creates convincing human-like speech, translate languages, answer questions, and more [2]. OpenAI tested whether people could identify 500-word fake new articles written by their model. Their results showed that people could only accurately identify the article was AI generated 52% of the time, which is concerning [2].

Writing news articles is just the tip of the iceberg when it comes to generative language models. These types of models are used by AI bots on social media to convincingly act as real human accounts. If you are worried about that, they are also being used to reverse engineer and detect the presence of bots in order to combat them [13]. With how fast improvements keep coming, these generative language models might be the main brain behind a general-purpose AI, helping it better understand real-world concepts to generally help the user.
Conclusion
While AI generated content isn’t going to take anyone’s job away, it will reduce some of the basic work creators endure such as, concepting and sketching out ideas [3]. Artists and creators will be able to do more with their time and be able to get help with menial tasks from the AI. Also, with the rapid development of these AI systems a pertinent question is the lack moral standing of these models. AI generated content could have tremendous power to influence culture and society, especially when it will be capable of creating an entire movie from scratch [14]. The lack of a mind (or soul as the artists said) is going to be a very important thing to keep an eye on as it could negatively influence society [14].
Artificial intelligence has advanced tremendously since the invention of generative models in 2014 and doesn’t seem to be stopping. The work being done on reinforcement learning today will have a huge impact on how fast this type of AI comes one day. Our dream of an AI driven future is already close to fruition. The image and art accomplishments will be the key to giving the general-AI it’s sight, while the progress in human-like speech generation will be the main brain behind it, to help better understand concepts for problem solving. With these models continuing to improve, it is almost inevitable a general-AI emerges from it.
REFERENCES
[1] H. Osipyan, B. I. Edwards, and A. D. Cheok, Deep Neural Network Applications, 1st ed. Boca Raton: CRC Press, 2022. doi: 10.1201/9780429265686.
[2] T. B. Brown et al., “Language Models are Few-Shot Learners.” arXiv, Jul. 22, 2020. Accessed: Jun. 24, 2022. [Online]. Available: http://arxiv.org/abs/2005.14165
[3] H. Zhan, L. Dai, and Z. Huang, “Deep Learning in the Field of Art,” in Proceedings of the 2019 International Conference on Artificial Intelligence and Computer Science, Wuhan Hubei China, Jul. 2019, pp. 717–719. doi: 10.1145/3349341.3349497.
[4] S. Tingiris and B. Kinsella, Exploring GPT-3. Birmingham: Packt Publishing, 2021. [Online]. Available: https://go.exlibris.link/y93YHH6M
[5] A. Alabdulkarim, W. Li, L. J. Martin, and M. O. Riedl, “Goal-Directed Story Generation: Augmenting Generative Language Models with Reinforcement Learning.” arXiv, Dec. 15, 2021. Accessed: Jun. 09, 2022. [Online]. Available: http://arxiv.org/abs/2112.08593
[6] A. Ramesh, P. Dhariwal, A. Nichol, C. Chu, and M. Chen, “Hierarchical Text-Conditional Image Generation with CLIP Latents.” arXiv, Apr. 12, 2022. Accessed: Jun. 20, 2022. [Online]. Available: http://arxiv.org/abs/2204.06125
[7] A. Ramesh et al., “Zero-Shot Text-to-Image Generation.” arXiv, Feb. 26, 2021. Accessed: Jun. 24, 2022. [Online]. Available: http://arxiv.org/abs/2102.12092
[8] I. J. Goodfellow et al., “Generative Adversarial Networks.” arXiv, Jun. 10, 2014. Accessed: Jun. 10, 2022. [Online]. Available: http://arxiv.org/abs/1406.2661
[9] “Minimax Algorithm in Game Theory | Set 1 (Introduction),” GeeksforGeeks, Jun. 14, 2016. https://www.geeksforgeeks.org/minimax-algorithm-in-game-theory-set-1-introduction/ (accessed Jun. 20, 2022).
[10] Z. Wang, Q. She, and T. E. Ward, “Generative Adversarial Networks in Computer Vision: A Survey and Taxonomy,” ACM Comput. Surv., vol. 54, no. 2, pp. 1–38, Mar. 2022, doi: 10.1145/3439723.
[11] A. Aggarwal, M. Mittal, and G. Battineni, “Generative adversarial network: An overview of theory and applications,” Int. J. Inf. Manag. Data Insights, vol. 1, no. 1, p. 100004, Apr. 2021, doi: 10.1016/j.jjimei.2020.100004.
[12] Y. Hong, U. Hwang, J. Yoo, and S. Yoon, “How Generative Adversarial Networks and Their Variants Work: An Overview,” ACM Comput. Surv., vol. 52, no. 1, pp. 1–43, Jan. 2020, doi: 10.1145/3301282.
[13] S. Najari, M. Salehi, and R. Farahbakhsh, “GANBOT: a GAN-based framework for social bot detection,” Soc. Netw. Anal. Min., vol. 12, no. 1, p. 4, Dec. 2022, doi: 10.1007/s13278-021-00800-9.
[14] G. Lima, A. Zhunis, L. Manovich, and M. Cha, “On the Social-Relational Moral Standing of AI: An Empirical Study Using AI-Generated Art,” Front. Robot. AI, vol. 8, p. 719944, Aug. 2021, doi: 10.3389/frobt.2021.719944.
No comments:
Post a Comment